Thank you to the Department of Justice for this opportunity to comment on the topics of the May 30, 2024 workshop, “Promoting Competition in AI.” I am the Head of AI Policy at the Abundance Institute, a new mission-driven nonprofit dedicated to creating an environment where emerging technologies, including artificial intelligence, can germinate, develop, and thrive in order to perpetually expand widespread human prosperity and abundance.
Competition is a key ingredient of this prosperity-inducing environment. Competition ensures that consumers face genuine choices in the market, providing useful feedback to firms. And competition spurs rivals to innovate.
But more importantly, innovation spurs competition. Consider the release of ChatGPT, a single webapp which became the most rapidly adopted technology ever, catapulted a previously unknown company into global fame, triggered a surge of investment, and shocked existing tech giants into action.
The result is one of the most dynamic, rapidly evolving tech ecosystems in history. FirstMark’s 2024 Machine Learning, AI & Data Landscape features 2,011 different company logos, “with 578 new entrants to the map” since last year, grouped into nine major categories and 100 sub categories.
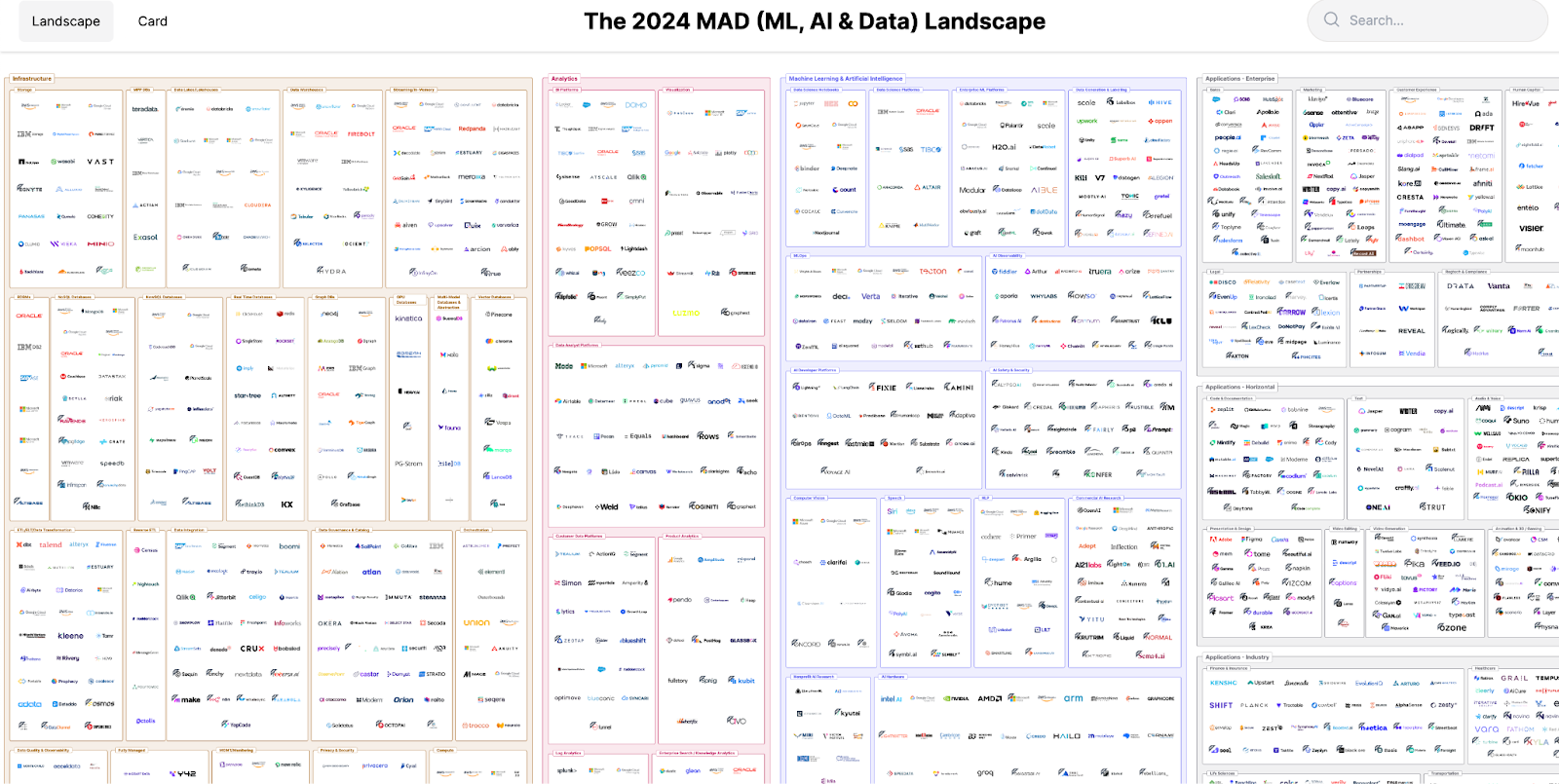
If competition agencies wish to probe beyond the obvious fact that this increasingly vast, complex ecosystem is vibrant in competition and innovation, how might they do so? Below, I discuss four key ideas regarding competition and artificial intelligence. First, the complexity of the AI ecosystem means that competition authorities ought to apply a dynamic competition framework to accurately understand and to guide any interventions. This approach has direct relevance for ongoing investigations. Second, preserving and promoting open-source development and deployment will strengthen the competitiveness of the AI ecosystem. Third, competition authorities should seek to ameliorate and prevent artificial, often government imposed barriers to competition – including those imposed by other countries. Finally, competition authorities should anticipate and facilitate the pro-competitive effects of increased interoperability that is likely to be a product of widespread use of AI tools like Large Language Models.
The Importance of a Dynamic Approach to AI Competition
The complex AI ecosystem calls for a new emphasis on dynamic analysis in the economics underlying antitrust law. A dynamic competition approach requires a deeper understanding of the AI ecosystem but promises results that more clearly reflect competitive realities. Absent such an approach, antitrust enforcers risk wasting money and harming competition.
The Complexity of the AI Ecosystem
AI is a general purpose technology – perhaps the most general purpose technology – meaning that AI has applications in all industries. AI will thus shape competition within every industry where it is applied. Often these applications will decentralize and enhance competition. For example, as increasingly powerful generative AI tools lower the barriers to creating high-quality content, expect smaller teams to better compete with larger, more-resourced incumbents. Market conditions or government interventions that reduce the availability of AI tools will limit this pro-competitive effect across industries.
However, most discussion in the workshop and among competition analysts has focused on analyzing competition within the AI industry. Even ignoring the broad application of AI, such analysis is challenging. Artificial intelligence tools are a complex “stack” of technologies. For example, an AI-powered smartphone application may use an application programming interface (API) to connect to an OpenAI ChatGPT instance running on a Microsoft Azure cloud service that is powered by custom networked computers using Nvidia hardware. Some AI company offerings span layers; others are more limited.
Each layer of the stack has a novel economic structure. For example, foundational models, as software, have significant returns to scale. Once trained they can be deployed many times, for many different uses, and the marginal user adds little additional cost. In contrast, the computational infrastructure – chips, computing clusters, even data centers – have more limited returns to scale due to the cost of manufacturing physical components.
Additional complexity comes from the fact that interventions or market changes to one of these layers has ripple effects across other layers. For example,
“[A] lack of competition at the infrastructure layer would certainly affect AI foundation models, but the agents at the layer of those models would respond by investing in infrastructures. This dynamic is already in play. OpenAI is reportedly trying to raise $7 trillion to develop its own chips and computing power. Aware of this risk, Nvidia is pushing to steadily lower the cost of training LLMs, from $10 million a few months ago to as little as $400,000…”
Simple competition analysis might separate layers of the AI stack into different markets, but any analysis that fails to consider these cross-layer currents would be incomplete.
Finally, the progress of AI technology continues to evolve and is difficult to predict. For example, the recent trend has been that models continue to gain capabilities primarily through scaling the number of parameters, driving continuously increasing demands for data and compute. But there is some evidence that scaling will soon fail to provide corresponding returns. An AI ecosystem without perpetual improvement through scaling looks very different, from a competition perspective, from one where scaling continues to return outsized value.
The Need for a Dynamic Competition Framework
The general purpose nature of AI, the different business models and economic incentives at various layers of the AI stack, the inherent feedback loops between the various layers of the stack, and the difficulty of predicting the technology trends mean that the AI ecosystem is highly complex and dynamic.
The static market paradigm that has typified economic analysis in antitrust cases is not well-suited to analyze this ecosystem. Instead, competition authorities should analyze the AI ecosystem through what renowned economist David Teece refers to as the “Dynamic Competition Framework.”
The Dynamic Competition Framework Improves on the Status Quo
Effective competition analysis must begin with understanding how firms compete. Broadly speaking, firms can compete two different ways. First, they can deploy competing cost-savings efficiency measures to deliver the same products or services to consumers at ever lower prices. Second, firms can compete to create new products, services, and business models that serve new needs or satisfy existing demand in new ways.
Standard antitrust analysis today focuses primarily on the first form of competition. It uses static competition models which evaluate efficiency within existing markets rather than anticipating innovation and future markets. According to Teece, static models envision competition as a simplistic version of reality where "existing products are offered by competitors at low prices” all of whom “have the same or very similar technologies or business models,” and where “markets are in a stable equilibrium.” In this framework, "no new products are introduced, and rapid price reductions driven by innovation do not exist." Static models tend to emphasize market shares, concentration metrics, and short-term price effects as key indicators of competition.
Static models “permit predictable modeling, but they sacrifice connections to competitive reality.” In other words, they are predictable but wrong in important ways. At best, use of these types of models in antitrust analysis can suggest how to improve the efficiency of existing markets and short-term price decreases for consumers. But even these beneficial outcomes can come at a cost. They nearly entirely disregard disruptive innovation, which is the “true handmaiden of competition” and the real driver of “long-term consumer welfare.”
To be sure, the status quo of economic analysis in antitrust has critics. Neo-Brandeisians, concerned primarily with the political power of large organizations, have also criticized applications of the static framework of analysis as overly obsessed with efficiency and short-term benefits. But their solutions would replace the too-simplistic economic analysis of static models with legal presumptions and structural rules that largely eschew case-by-case economic analysis. Their preferred approaches would thus be exercises of political power largely indifferent to reality’s complexities.
There is a path to better align antitrust policy with innovation: the dynamic competition framework (DCF). DCF is a sustained and ongoing effort to create a more comprehensive and realistic model of competition that can address the complexities of modern, innovation-driven economies. It challenges traditional antitrust economic analysis by arguing that current practices often underestimate the role of innovation and overemphasize static market structures. Drawing inspiration from the Austrian school of economics and Joseph Schumpeter's concept of “creative destruction,” the framework has been cultivated by Teece and other scholars across several disciplines, including organizational economics, strategic management, entrepreneurship, and innovation studies. DCF incorporates principles from evolutionary and complexity economics while emphasizing the role of management, business models, and strategy in competition analysis.
Key elements of the Dynamic Competition Framework include:
Innovation-Driven Competition: The framework prioritizes innovation as the primary driver of competition, rather than static efficiency. It posits that innovation drives competition at least as much as competition drives innovation.
Capabilities-Based Analysis: The framework emphasizes the importance of firm-level capabilities, including ordinary, super-ordinary, and dynamic capabilities. These capabilities are crucial in understanding a firm's competitive position and potential.
Forward-Looking Perspective: Unlike static models, this framework adopts a prospective view, considering potential future market developments and competitive threats.
Ecosystem Approach: Instead of narrowly defined relevant markets, the framework considers broader competitive ecosystems, including complementary products and services.
Long-Term Consumer Welfare: The framework advocates for a long-run consumer welfare standard that encompasses innovation, product availability, and quality improvements, not just price effects.
Supply-Side Focus: DCF calls for a deeper analysis of supply-side factors, including technological and organizational capabilities, both present and future.
Potential and Nascent Competition: The framework places greater emphasis on potential and nascent competition, arguing for a more nuanced approach to assessing competitive threats.
Mergers and Acquisitions: DCF provides a new lens for evaluating M&A activity, considering how transactions might enhance innovation and capabilities rather than just market concentration.
Disruption and Renewal: The framework recognizes both disruptive innovation and organizational renewal as key aspects of dynamic competition.
Multidisciplinary Approach: DCF draws on insights from strategic management, organizational behavior, and innovation studies, advocating for a broader analytical toolkit in competition policy.
Research continues on how to operationalize DCF in antitrust investigations and enforcement. But I believe antitrust authorities have been presented with a false dilemma when it comes to innovation: They’ve been asked to stick with the status quo or to return to a past era of antitrust. There is a much better third option: advance economic understanding in a way that aligns it more with the realities of the modern world. Rather than return to out-of-date practices and ignore what we’ve learned from economics, DCF seeks to push economics forward to better understand and analyze the modern world. The complexity of the AI ecosystem demonstrates the need for a new approach. AI provides a genuine opportunity for competition authorities to improve the economic analysis that underlies modern antitrust enforcement.
Innovation Drives Competition, as ChatGPT Shows
One of the above-listed key features of DCF is particularly relevant to the DOJ’s inquiry here. Much of the discussion at the May 30 workshop was framed as how competition can spur innovation. But “[t]he focus should rather be on how innovation, whether based on deep tech or business model innovation, affects competition.” As Teece explains:
“Although competition authorities and economists correctly recognize that competition drives innovation, they have not sufficiently appreciated that causation also runs the other way. Indeed … innovation (and what I will call ‘dynamic capabilities’) drives competition at least as much as, and probably more than, competition drives innovation.”
For example, consider the disruptive threat that ChatGPT and similarly structured AI chatbots pose to Google web search. Google’s flagship search product which is under antitrust scrutiny, produces a series of relevant links which search users can visit to get the answer they seek. The format of providing options for the user is essential to Google’s search product. The choices that users make between those links are critical feedback into Google’s ranking algorithms. The “user-picks” format is also what enables Google to sell search advertising.
By contrast, consumers using AI chatbots receive a single answer to their query. In many ways, this is more convenient for the user. But single answer solutions lack a feedback loop for the search algorithm and offer no natural opportunity to integrate ads. Google has experimented with delivering answers in a similar single-answer format using its “AI Overviews” services. But where do ads fit this model? And how does it gather user feedback to improve? This service would appear to cannibalize the user attention that it previously sold to advertisers.
That innovation can and does drive competition has two implications for competition authorities. First, competitive analysis of a market is flawed if it fails to consider the potential of disruptive entry through innovation. Second, competition can be increased by promoting innovation through other, non-antitrust means. Third, mistargeted antitrust enforcement that prevents innovation can prevent the emergence of disruptive competitors, ultimately undermining competition.
Evaluating Acquisitions, Investments, and Contractual Arrangements by Large AI Companies Under DCF
DCF analysis could be useful in certain ongoing investigations in the AI ecosystem. Competition authorities are reviewing mergers, investments, and contractual arrangements between large technology companies and newer AI firms. Under antitrust law such arrangements are usually evaluated under the rule of reason, to weigh their procompetitive benefits against the anticompetitive harms.
DCF would bring different evidence to this analysis by emphasizing the importance of innovation and capabilities in assessing the competitive effects of such arrangements. Market shares and concentration ratios are only part of the story. Agencies should evaluate the involved parties' technological and organizational capabilities, both present and potential, to understand the true competitive landscape. They should gather the information necessary to evaluate the complementarity of the merging firms' capabilities and their potential to drive systemic or architectural innovation. They ought also assess the likelihood of supply-side responses and potential competition more thoroughly, taking into account the fungibility and adaptability of firms' capabilities.This is not necessarily a free pass for such arrangements. A forward-looking perspective would consider how a merger might enhance or impede innovation and dynamic competition in the relevant ecosystem.
In evaluating mergers involving nascent or potential competitors, DCF analysis would allow that nascent firms often fail to develop into mature and able competitors for reasons that have nothing to do with their market rivals. External investment, even by large incumbent firms, can better enable that maturity. And acquisition is a viable path to the widespread implementation of an innovation where the innovating company lacks the management and other capabilities to grow on their own.
But some such acquisitions are problematic. Teece has proposed a set of criteria to determine when nascent competitor acquisitions might pose genuine competitive concerns:
1. The acquiring firm has monopoly power.
2. The nascent firm’s technology has passed proof of concept (i.e., the technology works).
3. The nascent firm has a proven business model to monetize the technology.
4. The nascent firm has an existing entrepreneurial leadership team and strong capabilities to carry the enterprise forward for at least 5–10 years, or has a credible succession plan in place.
5. The nascent firm’s technology will be disruptive to core revenue streams of the acquiring firm.
6. The technology of the nascent firm is not competency- enhancing (complementary) to the acquiring firm. Rather, it’s primarily competency-destroying and, hence, threatening.
7. There are no other nascent competitors similarly situated.
A similar criteria could be applied to analyze investments and contractual arrangements. (Acknowledging, of course, that investments and contractual arrangements do not eliminate a market participant and therefore are reviewed under a different set of legal criteria.)
The DCF approach requires a deeper factual inquiry and a more nuanced understanding of industry dynamics, technological trends, and organizational capabilities. There are indeed challenges to operationalizing this framework, but I believe it offers a more accurate and relevant basis for analysis in innovation-driven markets.
***
These are only some of the many lessons of the dynamic competition framework for AI. I highly recommend reading the entirety of David Teece’s “The Dynamic Competition Paradigm: Insights and Implications,” for further lessons about how antitrust analysis can adapt to better identify competition opportunities and challenges in the complex AI ecosystem.
Open Source is a Key Vector of AI Competition
Open-source software has long been an enabler of competition within software development. As I and my co-authors noted in comments to the National Telecommunications and Information Administration, this is also true in the artificial intelligence ecosystem. Open model weights affect competition both in the marketplace for AI services and in other areas of the economy in many ways, including:
Leveling the playing field: Open models reduce the barriers to entry and give smaller players and startups access to cutting-edge AI technology. This could increase competition across the economy as more organizations are able to leverage powerful AI capabilities in their products and services without needing the massive resources to develop the foundational models themselves. This leveling effect is supported by research that demonstrates that using generative AI tools in work settings disproportionately benefits lower-performing workers.
Shifting focus to applications and fine-tuning: With shared access to strong open models, competitive differentiation will depend on how well companies can adapt and apply the models to specific domains and use cases. The ability to efficiently fine-tune models and develop powerful applications on top of them could become more important than the ability and capacity to train a foundational model from scratch.
Commoditization of foundational models: In the long run, open models could commoditize foundational AI technology. If everyone has access to high-quality open models, the models themselves may not be a sustainable competitive advantage. The real value may migrate to compute, proprietary datasets, customizations, and application-specific IP. This would distribute gains from this technology more broadly across the economy.
New business models: Open models could spur new business models and ways of creating value in the AI ecosystem. For example, there may be opportunities to provide compute resources for fine-tuning, offer managed services around open models, or develop proprietary add-ons and extensions.
Collaboration and shared standards: Open models could foster greater collaboration and interoperability within the AI community. Shared standards and a common technological substrate could emerge, enabling more vibrant competition in the application layer.
Quality and safety assurance: With open models, there may be more intra-firm competition to ensure the quality, safety, and responsible use of foundational models. Expertise in AI alignment, safety, robustness, and ethical deployment could become key competitive differentiators, both within open-source and proprietary models.
The primary implication of all of these points is that open models do and will increase competition at the model layer, spurring innovation at that layer and distributing value creation to other layers of the AI stack. I applaud FTC staff for a recent statement recognizing the pro-competition and pro-innovation benefits of open-source AI, and Chair Lina Khan’s online commentary echoing those sentiments. Competition agencies ought to continue to support open source as a powerful avenue of competition and innovation.
Removing Artificial Barriers to Competition
Another way that competition authorities could enhance competition in the AI ecosystem is by advocating for the removal of artificial barriers to AI innovation, many of which are government imposed.
For example, building on the discussion in the previous section, regulatory restrictions that harm the development or distribution of open-source AI or open weight models can be expected to harm competition. Competition authorities ought to weigh in on regulatory approaches to AI, favoring those that preserve and promote the ability of open-source models to flourish and expand. The FTC in particular has a long history of competition advocacy and ought to consider providing state legislatures guidance on how to develop AI regulatory approaches that do not impinge on open-source development and usage.
In addition to potential threats to open-source AI, there are other existing regulatory constraints on AI competition, and many more being considered. These include state-level AI regulations with high compliance costs likely to lead to capture by the largest players, overly restrictive data regulation regimes that limit the collection of useful information (again favoring incumbents with substantial amounts of data), and copyright structures that deter constitutionally-protected fair use. Any serious evaluation of how to promote competition in AI must consider how legal and policy restrictions affect such competition.
Finally, not all existing or expected artificial barriers to vibrant AI competition are domestic. Some regions – the European Union in particular – have adopted laws with intentionally global effect and have historically demonstrated an enforcement focus on U.S. companies. Such actions should be seen as protectionist and potentially anticompetitive, and the U.S. Government should use its diplomatic and trade authority to preserve vibrant competition in AI.
Increased Competition Through AI Interoperability
Competition authorities should be aware that there is one specific application of recent AI innovations that could increase competition across the software industry: large language models (LLMs) could turbocharge interoperability, breaking down barriers between existing software and increasing the dynamism of the software ecosystem.
Interoperability allows different systems, devices, software applications, or services to communicate, exchange, and effectively use information with one another. It often requires adhering to common standards, protocols, or interfaces. This can improve user experience, streamline workflows, and reduce costs.
In the policy sphere, interoperability is frequently sought as a remedy to competition concerns such as lock-in and monopolization.
Cory Doctorow has described a taxonomy of interoperability, with three categories: cooperative, indifferent, and adversarial. LLMs offer new possibilities for indifferent and adversarial interoperability and will even enable a new era of automated, dynamic cooperative interoperability.
Cooperative interoperability is a common approach in software, typically through the use of Application Programming Interfaces (APIs). These APIs provide a predefined set of rules, functions, and protocols that allow developers to create software that can interact with other software. Organizations like the World Wide Web Consortium (W3C) establish standards for web-based interoperability, while industry-specific initiatives, such as the Fast Healthcare Interoperability Resources (FHIR) standard for healthcare, have emerged to address the unique challenges within various sectors.
Cooperative interoperability is difficult and time consuming because parties can have unaligned interests. Even once established, standards are necessarily brittle and static. If you modify an API to add or change functionality, you might break all the software that uses it.
LLMs like ChatGPT can facilitate indifferent and adversarial interoperability by serving as "universal APIs" between different user interfaces. User interfaces are the parts of software that we humans interact with. They employ textual and graphical languages that we can interpret - and such interfaces are part of the content that modern LLMs have been trained on.
LLMs can easily translate such user interfaces into more formal, API-like code. This capability allows on-the-fly creation of APIs for any service with a user interface. A web browser with LLM capabilities could, for example, interact automatically on your behalf with Facebook or Twitter through the same user interface that you would use. LLMs could even provide connection between services similar to what Zapier and IFTTT do - but unlike those services, they could work even when the software services being connected offer only a user interface, with no formal API exposed. Perhaps most usefully, if a webpage or service changes its user interface, LLMs would be able to adjust without the need for a human to rewrite the API.
The potential competitive implications of LLM-based interfaces between different pieces of software include:
Less Risk of Standards-Based Market Power. Rather than relying on standard-setting bodies, which can be dominated by large intellectual property holders, future software systems could negotiate how to communicate through an iterative and dynamic process starting at a natural language level but quickly evolving a more specific, efficient, built-to-purpose interface. This would also mitigate the legacy support problem of cooperative interoperability APIs, making it less necessary to standardize.
Entry Through Adversarial Interoperability Becomes Technically Easier. Adversarial interoperability will become easier to do and harder to prevent. LLMs will be able to read and interact with the same interface humans use. If a service provider attempts to break the interface for LLM use, he will probably break it for users, too. New startups who wish to leverage incumbent services will be technically capable of doing so without seeking permission from the incumbent. Incumbents will become more dependent on legal and contractual restrictions to maintain the walls around their gardens.
Interoperability as a Data Source. Vendors offering API-like translation services would have a bird’s eye view of an ecosystem of platform-to-platform interactions. Such services would be well-positioned to learn general principles on how to better mediate communication between different pieces of software, evolving and becoming more efficient over time. A centralization of such services could enable enormous modularity and flexibility elsewhere in software services.
No Need for Interoperability Mandates. Advocates for interoperability often turn to regulation to overcome the business incentives and coordination costs that can prevent cooperative interoperability. To the extent that LLMs ease the practical difficulties of developing APIs and make it more difficult to block adversarial interoperability, government mandates become less necessary.
U.S. competition authorities should closely consider how they can support the emergence of LLMs as pro-competitive tools of interoperability.
Conclusion
In short, I urge competition authorities, including the Department of Justice, to consider the following factors when seeking to address competition in AI markets.
Competition doesn’t just drive innovation; innovation can also drive competition.
The complexity of the AI ecosystem requires a dynamic competition approach for understanding and intervening in markets.
Preserving and promoting open-source development is vital for maintaining competitiveness in the AI ecosystem.
Competition authorities should work to remove artificial, often government-imposed barriers to competition in AI.
Large Language Models can significantly increase interoperability across the software industry, potentially enhancing competition.
Thank you for the opportunity to share these perspectives.
Note
The Abundance Institute is a mission-driven non-profit dedicated to creating the policy and cultural environment where emerging technologies can develop and thrive in order to perpetually expand widespread human prosperity. This comment is designed to assist the agency as it explores these issues The views expressed in this comment are those of the author(s) and do not necessarily reflect the views of the Abundance Institute.