“Democratizing” AI is like “democratizing” a tank. Sure, we can make it open-source … or even let civilians become tank commanders. But a tank is still a tank.
– Evgeny Morozov, technology critic
Having AI servants will make everything easier for adults. Having AI servants will make everything easier for children, too, who will then not learn to do anything hard.
–Jonathan Haidt, social psychologist
Abstract
The growth of new technology, in particular new communication technology, has raised questions about technology’s role in society. Critics argue that it has increased hate speech, polarized the electorate, reduced deliberation, and coarsened the discourse. Others have emphasized the democratizing potential of tools facilitating collective action and the potential for new exchange of ideas. To better understand citizens’ general orientation toward technology, we develop a new anti-technology scale and test it on two diverse samples of Americans. Our scale measures three distinct areas of anti-technology attitudes: 1) attitudes toward social media, 2) attitudes toward artificial intelligence, and 3) concerns about modernity. We show that these areas form a general, latent anti-technology orientation. We then show that this general anti-tech orientation predicts attitudes toward technology policies and support for contentious actions against tech companies. Finally, we use a pairwise comparison experiment to understand which pro- and anti-AI arguments are most persuasive.
Introduction
Concerns about new technology, similar to those expressed by Morozov and Haidt, are long-standing. Many observers believe that technology makes users cognitively lazy and increases mental health problems. Other experts have warned that the recent arrival of powerful large language models (LLMs) like ChatGPT, and future increased capabilities of artificial intelligence (AI), will have negative consequences for workers by taking jobs, and will increase misinformation. Pew Research polls further show that Americans are more “concerned” than “excited” about AI, and YouGov survey data examined by the AI Policy Institute shows that 60 percent of Americans feel “AI will undermine meaning in our lives by automating human work, making humans less useful and relevant, and weakening our social bonds.” A growing chorus of voices argues that the business models or products of digital tech are fundamentally at odds with liberal democratic values. In addition, media and business personalities with a large following, such as Elon Musk and Tucker Carlson, have spoken favorably about Ted Kaczynski, the Unabomber and author of an anti-technology manifesto.
Although various risks of AI are currently speculative, arguments that social media use is dangerous for children and teenagers and that social media companies prioritize profit over the safety and well-being of its users have resonated with citizens for some time. Recent public opinion polls reflect this antipathy, showing a decline in trust toward social media companies, with Americans increasingly concerned about surveillance, misinformation, and the cyber risks posed by the increasing use of technology. A survey on confidence in institutions and support for democracy looked at different American companies and institutions between 2018 and 2021, and found that tech companies such as Facebook, Amazon, and Google experienced the steepest drop in trust. While scholars disagree about the impact of dubious or fake content on voters, most experts believe social media has exacerbated the spread of misinformation.The growing concern over the impact of technology on society raises the question: what determines attitudes toward technology? In this paper, we measure anti-technology sentiment among Americans, test its predictive power in explaining policy preferences and contentious political behavior, and discuss its political implications.
Previous research has focused on individual attitudes toward specific technologies such as social media, artificial intelligence, and robots, as well as on people’s fluency or comfort with technology. Researchers have also investigated trust in Western medicine and explored whether trust in folk medicine predicts anti-expert attitudes. Further research finds that anti-tech sentiment, particularly toward social media companies, is not a solely motivated by partisanship: large majorities of both Democrats and Republicans feel that social media companies have too much power and influence, believe “Big Tech” is a problem for the US economy, and favor breaking up the biggest tech companies. However, existing research does not distinguish whether these various components of anti-technology attitudes are distinct or represent a shared anti-technology orientation.
Previous research has documented the widespread economic benefits of new technologies on standards of living and welfare. But technology does not just influence the economy. It also influences culture and society, a core issue for much of sociology and political science. Modernization and associated theories argue that shifts in technology can help countries become wealthier and more advanced, and more likely to become democracies. Thus, new technology provides a virtuous cycle of more wealth, more stability, and more democracy. Yet other sociological theories argue that advances in technology can lead to a breakdown of social ties, create disorder, and foster alienation. These competing effects of new technology are at the heart of the tension between modernization theories and sociological theories.
All of this suggests three things about anti-technology sentiment: 1) that it is an important and possibly growing phenomenon; 2) that it is core to many important political and sociological theories; but 3) that it has been undertheorized and frequently assessed using limited metrics.
Using a pair of surveys and an experiment, we take an empirical approach to measure the contours of anti-technology sentiment among Americans. First, we measure three distinct anti-technology components: 1) negative attitudes toward social media , 2) fears over AI, and 3) a negative view of modernity. We show that these anti-technology components form an anti-tech disposition, which is relatively common among Americans. For instance, a majority of our survey respondents agree that “technology has taken over our lives” or that social media harms young users and fuels envy and social comparisons. Over half of respondents also fear that AI could hurt humans. Psychological variables including loneliness and conspiracism are associated with stronger anti-tech sentiment. Partisanship plays a modest role as well (skepticism of technology and modernity is marginally more common among Republicans than Democrats). Unlike partisanship, the anti-tech orientation predicts support for breaking up big technology companies. We also find a positive association between anti-tech attitudes and endorsing or excusing (hypothetical) extremist anti-tech behavior. Finally, using a pairwise survey experiment, we show that positive and negative sentiment toward AI is malleable.
In doing so, our paper represents a new and important contribution. Surveys that have previously focused on technology usually focus on a few key flavors of anti-technology (e.g., social media or AI) or negative implications of new technology (e.g., job losses). We take a comprehensive approach to anti-technology attitudes and explore their structure and implications.
Having established the context and importance of anti-technology sentiment, we now turn to our theoretical framework and expectations. Drawing from existing literature on social stress, political alienation, and technological change, we propose several hypotheses about the nature and consequences of anti-tech attitudes.
Theoretical Expectations
What explains core attitudes toward technology? Central to this question are the social effects of new innovations. Technological advances can create social stress and upheaval. Others argue that new technology is a form of social control. New technologies create new markets and upend economies, reshaping society. For instance, the data-driven economies of the web have individual companies competing for individual eyeballs and attention, all while consumers provide their own data in exchange for access to the new media applications. As Acemoglu and Johnson argue, new technologies generate new wealth and advances, but it is not uncommon for elites to capture these benefits, leading to social and economic marginalization for ordinary citizens. So, while new technologies lead to more wealth and increase standards of living, they also create new winners and losers and, subsequently, social tensions.
New communication technologies have always engendered critics. For instance, in the 1970s, prominent environmental and anti-technology activist, Jerry Mander called for the abandoning of television, believing it hopelessly irredeemable. He famously said, “To speak of television as ‘neutral’ and therefore subject to change is as absurd as speaking of the reform of a technology such as guns.” Similar critiques have been leveled against social media, with some arguing that its underpinnings of social validation, virality, and polarizing content are not only addictive but also harmful—to human interactions generally and to young adolescents and teenagers especially. Others suggest that social media, while potentially helping activists and the voiceless organize, also can polarize the populace, lead to echo chambers, facilitate the worst political impulses with hate speech, and empower authoritarian governments.
The arrival of new technology can also increase political alienation. This occurs when individuals feel isolated, lonely, angry at the status quo, and estranged from the current political system. Conspiracism and anti-establishment politics are important side effects of alienation, currently forming a cleavage in American politics, which is separate from the left-right ideology and orientation. And several studies show how feelings of alienation, conspiracism, and anti-establishment politics are related to support for political violence. Some researchers find that the economic dislocation associated with automation can also influence voting behavior, while others, find limited effects of automation threats on political preferences. Perhaps most concerning is that automation effects of AI could greatly increase inequality and thus corrode democracy.
In sum, as new technology increases social stress and upheaval, certain people may be skeptical of the benefits of this new technology. And, we argue that there is a connection between new technology, political alienation, and anti-system politics. These observations form the basis for our preregistered hypotheses.
We focus on four questions in particular. First, is there a general anti-technological predisposition? In other words, do Americans blame technology for specific social problems, including nasty politics, loneliness, erosion of privacy, or the circulation of hate and misinformation? Second, what are the correlates of this anti-tech orientation? Are lonely individuals and those who harbor resentments against “the system” more critical of technology in general? Third, are people who score higher on this anti-tech orientation more likely to support regulation on technology companies and to approve of citizen-led violence against representatives of such companies? Lastly, which arguments in favor of AI adoption (if any) are viewed by citizens as persuasive?
Our expectations are as follows:
→ A general anti-tech orientation exists and will emerge from a factor analysis of questions related to concerns about social media, fear of AI, negative sentiment about modernity, and unfavorable or skeptical attitudes toward Western medicine.
→ Higher scores on the anti-tech orientation will be associated with loneliness and conspiracism.
→ Anti-tech orientation attitudes will predict support of regulatory policies (independently of partisanship).
→ Respondents who score higher on the anti-tech orientation will be more forgiving of a hypothetical violent act or perpetrator. In addition, approval of violence against leadership of a tech company will be higher among respondents who exhibit higher need for chaos relative to those with lower anti-systemic views.
Data
In July 2023, we conducted our first survey, recruiting respondents via the Lucid/Cint platform and collecting responses from a nationally diverse sample of seven hundred US adults (dataset 1, fielded July 24–25, 2023). We followed up the first study with a nationally representative poll in collaboration with YouGov (dataset 2; N=1,350, fielded between September 26 and October 2, 2023). In the first survey, we fielded a larger number of anti-tech constructs and their correlates to explore the contours of the anti-tech scale. The second survey is slightly more parsimonious (and contains nineteen questions tapping into respondents’ technology-related attitudes). Our research questions and selected expectations were preregistered before fielding the first survey.
Our respondents were presented with a series of five-point (strongly disagree to strongly agree) questions that aimed to gauge their attitudes toward technology. These questions were grouped into four main clusters in the pilot study: 1) evaluations of social media platforms’ effects on society, health, and politics; 2) fear or optimism about AI; 3) views on modernity; and 4) questions capturing perceptions about the efficacy and trustworthiness of Western medicine versus alternative medical practices. The pilot study confirmed that the “Western versus Traditional Medicine” set of questions represented a separate factor from the remaining questions, and questions about Western medicine were therefore not asked on the YouGov survey, given our main focus on the anti-technology attitudes.
The list below summarizes the questions we posed to our respondents:
Social Media (eight questions): This category aimed to capture respondents’ perceptions of the impact of social media on society and on themselves. For instance, respondents were asked to consider statements such as “Social media has mostly been a bad thing for society because it spreads hate and misinformation” and “The reason our politics is so nasty is because of social media.”
Artificial Intelligence (six questions in the YouGov dataset, four questions in the Lucid dataset): This segment probed respondents’ perspectives on AI and its implications. Sample questions from this category include the following: “I am worried that scientists are designing computer programs that could hurt humans;” “Computers and machines can help us do tasks that are too boring for humans to do;” “Artificial intelligence should not be allowed in schools;” and “The extinction of the human race due to AI is a real possibility.”
Modernity (five questions): Here, we sought to understand the sentiments toward the broader theme of modern technological advancements and their implications on human intelligence, behaviors, and lifestyles. Examples from this section include the following: “Our reliance on machines and technology has made us less smart;” “All this modern technology prevents us from living in harmony with nature;” and “We have let modern technology like smartphones take over our lives.”
Western versus Traditional Medicine (four questions; Study 1 only): Respondents were presented with statements such as “I trust alternative medical practices like homeopathy, acupuncture, Reiki, and herbal supplements more than modern Western medicine” or “Big pharmaceutical companies are secretly hiding cures for diseases like cancer.”
The full set of nineteen close-ended items we asked in the YouGov survey and the distribution of responses is displayed in figure 1. The wording of all questions is provided in the supporting information appendix (SI), and the distributions of responses from dataset 1 are shown in figure S.3.
The components of the theorized anti-tech attitudes are also listed in table 1, with checkmarks indicating whether a given set of variables was included in a specific dataset. The table also contains the main independent variables that were measured and indicators of their inclusion across surveys.
Outcome Variables
In addition to quantifying the prevalence and structure of anti-technology attitudes, our second objective is to explain policy preferences and views on politically controversial innovations such as facial recognition (when used by the police) or algorithms deployed by social media platforms to identify false information. We measured preferences by posing direct prompts to our respondents:
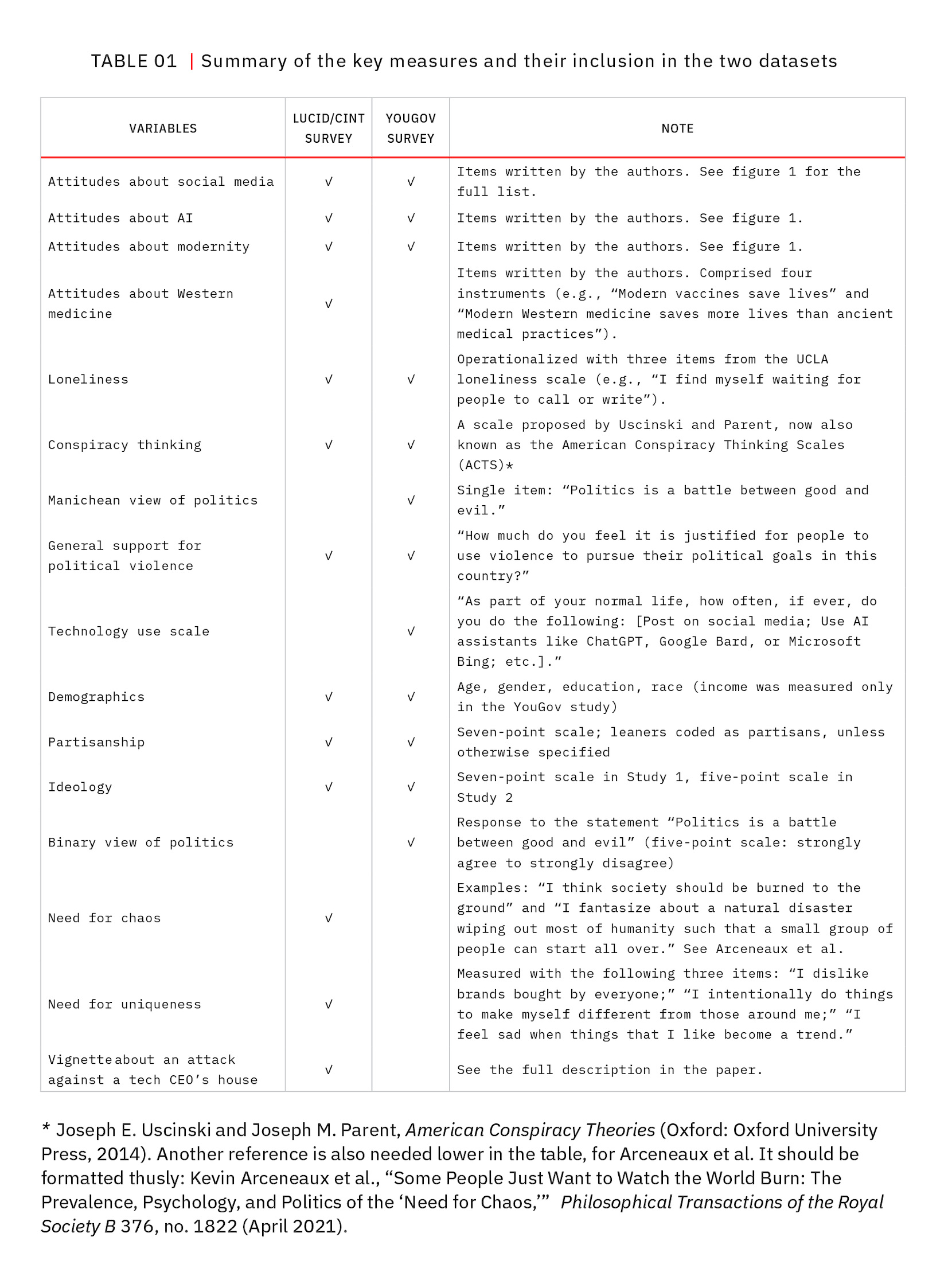
On regulating AI: “How much do you agree or disagree with the following statement? Research on artificial intelligence should be heavily regulated by the government.” (Scale of 1–5: strongly agree to strongly disagree)
On breaking up Big Tech: “Some people think that big tech companies like Google, Amazon, Apple, Meta/Facebook, and Microsoft are too powerful and should be broken up, while others say that big tech companies should not be broken up because it will hurt innovation and harm customers. Which comes closer to your views on big tech companies?” (Binary response scale: “Break up Big Tech” or “Do not break up Big Tech”)
The following is a list of new potential technologies. Would the widespread use of these technologies be a good or bad thing for society? (Binary response: “A good idea for society” or “A bad idea for society”)
→ Facial recognition by the police to spot criminals in crowds
→ Automated computer programs by social media companies to find false information
→ Robots that replace cashiers, cleaners, and cooks
→ Cars that are completely operated by computers and don’t need humans to
drive
→ A computer chip implanted in the brain that allows people to process information faster and more accurately
Next, we wanted to understand if, and to what extent, certain technological apprehensions may translate into support, sympathy, or excusing of extremist anti-tech views and actions. To that end, the following outcome variables (measured in dataset 1 only) aimed to measure evaluations of the views and actions of an anti-tech terrorist, who was described with the following vignette:
A man threw a firebomb into the empty house of a powerful billionaire CEO of a social media technology company. When asked why he did it, the man said, “Somebody had to do something. These tech CEOs knew that their social media apps divide our country and hurt our children. They don’t care. They just want to make money.”
After reading about the hypothetical scenario, respondents were asked to answer the following set of questions:
On support for violence: “How much do you support or oppose the man’s actions?” (Scale of 1–4: strongly oppose to strongly support, with no neutral option provided)
On agreement with the letter: “Regardless of whether you agree or disagree with the man’s actions, how much do you agree or disagree with what the man said?” (Scale of 1–5: strongly agree to strongly disagree, with a neutral option provided in the middle)
On punishment severity: “How much time in prison, if any, do you think the man should face?” (Eleven options provided, ranging from “No time in prison” to “More than twenty years in prison”)
The modal response to the punishment severity question was “2–5 years in prison,” and approximately 20 percent of respondents indicated that the perpetrator should serve at most thirty days in prison (this proportion includes the 9 percent of respondents who told us that the man should face no time in prison).
Results
We now present the findings from our surveys. Our analysis proceeds in four parts. First, we examine the structure and prevalence of anti-tech attitudes among Americans. Second, we investigate the correlates and predictors of these attitudes. We then explore how anti-tech sentiments relate to policy preferences and support for contentious political actions. Finally, we report results from our persuasiveness experiment.
Study 1: Anti-tech Attitudes
Structure of Anti-tech Attitudes
Our analysis begins by presenting the distribution of responses to the 19 tech-related questions which we asked in the nationally representative YouGov survey, as depicted in figure 1. What immediately stands out from the data is that tech-critical sentiment among respondents is common: significant majorities believe that social media has detrimental effects on children and teenagers, and there is a prevailing notion that modern technology on the whole has excessive influence over our daily lives. The perception that AI advancements will lead to job losses is also widespread. At the same time, most respondents also concurred that modern technology made their lives more convenient, and that social platforms facilitate activism or staying connected to one’s friends and family.
Exploratory factor analysis was used to discern if the meaningful clusters within our dataset were grouped by topics or some other attribute. The only substantive topic that emerged as a separate factor was the set of views of modern versus traditional medicine, measured in the initial survey. In the Lucid dataset, we identified three distinct groupings of variables: first, the dominant factor which emerged—and one we will rely on heavily throughout our analysis—encapsulates general anti-technology orientation. This factor gives us insights into the overarching sentiments of respondents toward AI, social media, and modernity in general. The second factor taps into skepticism of the tangible benefits derived from technology (e.g., respondents disagreeing that social media is a powerful tool for activism, or disagreeing that modern technology made their own lives more convenient).
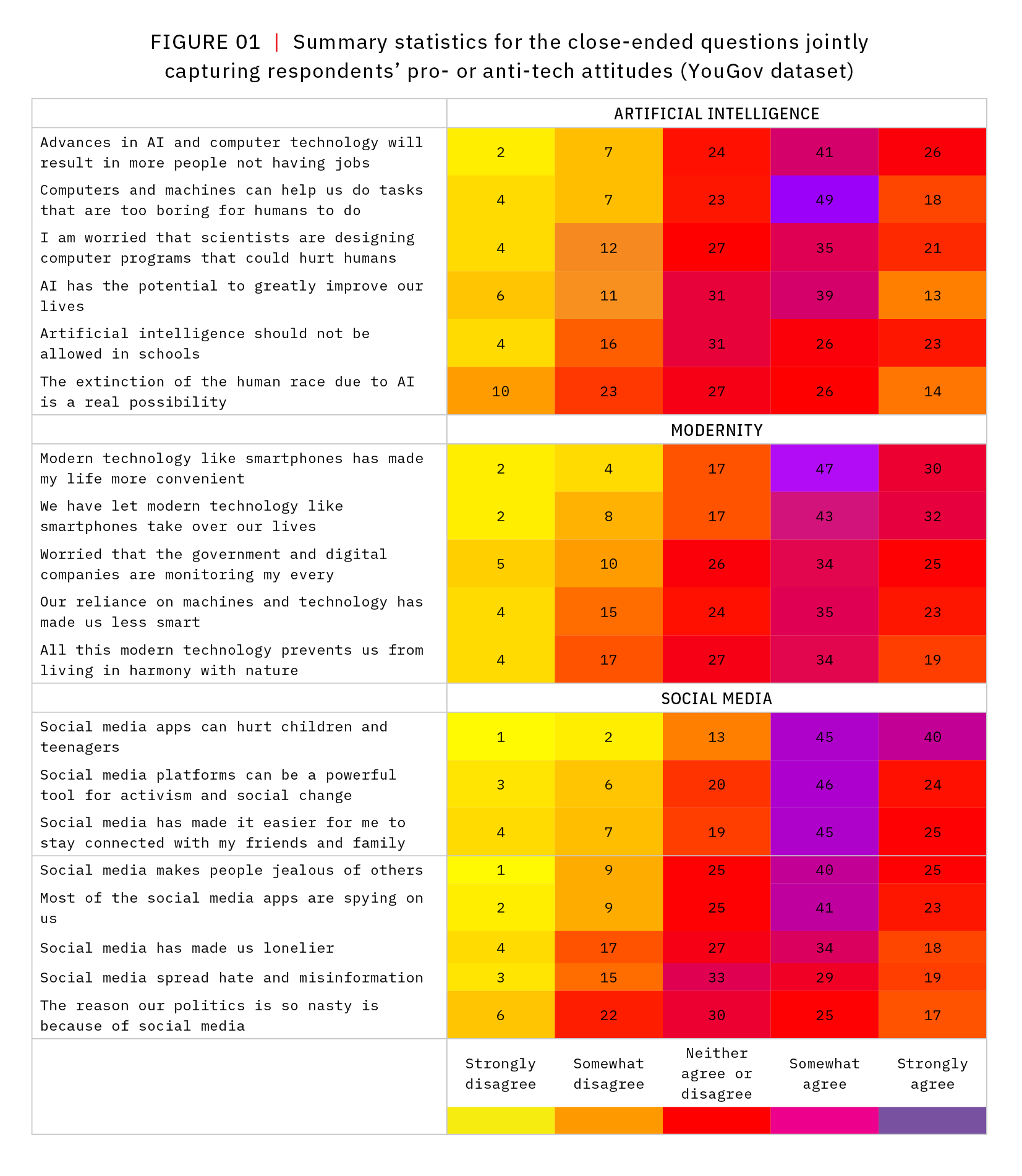
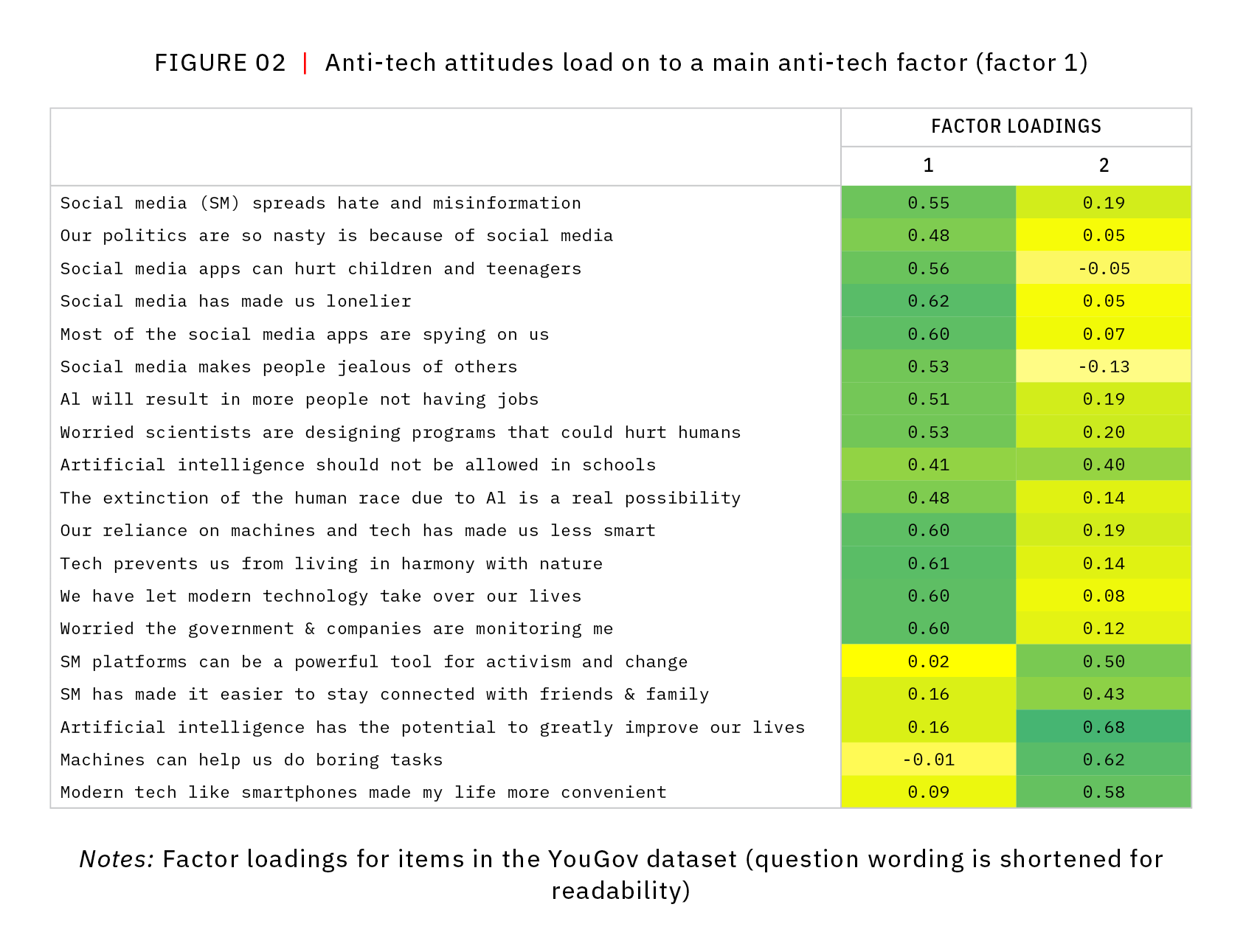
Meanwhile, the third factor emerged as a preference for traditional folk medicine blended with a skepticism of Western medicine practices (see figure S.4 for factor loadings among the Lucid respondents). Given these initial findings among our Lucid respondents, we posed the same set of questions about social media, AI, and modernity (plus two new items about AI) to YouGov panelists two months later to assess whether the emergence of a first dominant anti-tech factor replicates. Figure 2 shows the results. We see that in general two factors emerge: a general anti-tech orientation (factor 1) and techno-optimism (factor 2). This general anti-tech orientation (factor 1) will be our main factor of focus because it explains 46.6 percent of the variance, its eigenvalue is 4.99, and the Cronbach’s alpha statistic using all nineteen items jointly is 0.85, indicating excellent reliability.
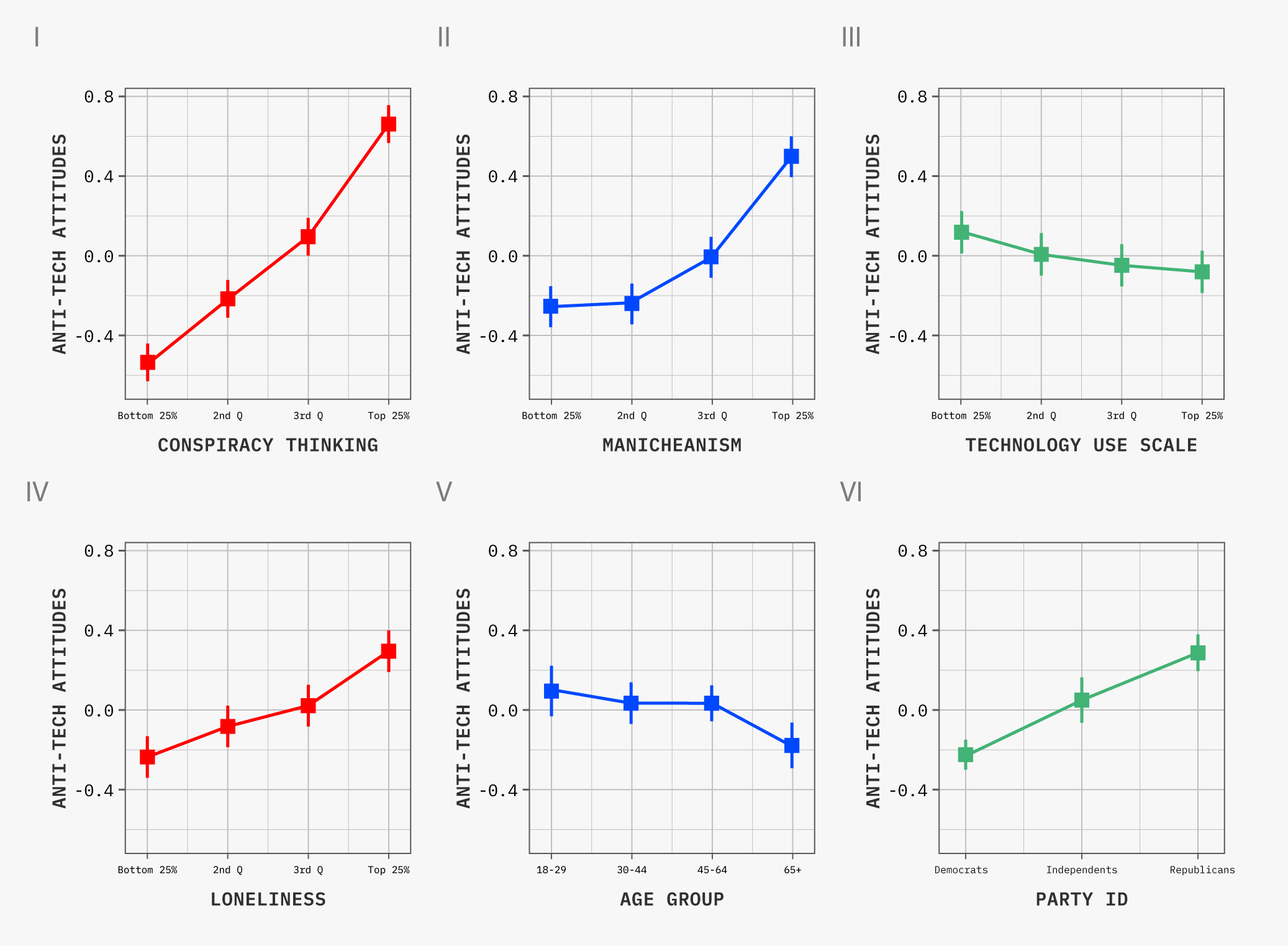
Correlates of Anti-tech Attitudes
Before turning to models of policy preferences or acceptance of innovations such as self-driving cars or facial recognition (where anti-tech attitudes will serve as the main independent variable), we first treat anti-tech attitudes as a dependent variable to better understand who is more likely to hold these attitudes. We start by plotting the distributions of these attitudes separately for different levels of the main covariates of interest.
Beginning with conspiracism in the top-left panel of figure 3, we find that (z-scored) anti-tech attitudes are 0.64 among the most conspiracy-inclined quarter of the respondents, and -0.54 among those in bottom quartile (i.e., the relatively pro-establishment respondents). As the slope in that panel indicates, the bivariate association between anti-tech attitudes and conspiracism is stronger compared to other potential predictors. The distance in anti-tech attitudes between the least lonely quarter of respondents and the most lonely quarter is 0.54 standard deviations (bottom-left panel of figure 3), which puts the 1.2 SD gap for conspiracism into perspective. We also see that people who use ChatGPT, smartwatches, social media, and smart home assistants are slightly more pro-technology compared to people who use these products less or do not use them at all (top-right panel in the same figure).
Perhaps surprisingly, the relatively older respondents express lower anti-tech attitudes: on average, Americans who are sixty-five years old or older score 0.16 below the mean level of anti-tech attitudes. We also see that Republicans are relatively more oriented against modern technology than Democrats (the slope is comparable to the slope we saw for the loneliness scale).
Another way to display unadjusted differences across groups of respondents is provided in figure 4. Here we also add participants from the Lucid survey to allow for a pooled comparison across variables of interest. The elevated levels of conspiracism, loneliness, and need for chaos indicate that the average level of anti-tech attitudes is high, and that the concentration of respondents with a strong opposition to technology is especially visible among these sets of respondents (top three rows of figure 4). Among people who identify as liberal, who do not feel lonely, or who are skeptical of general conspiratorial narratives, the average level of anti-technology attitudes is low (with the median ranging from -0.52 to -0.18), and the distribution around the median point is more symmetric.
But are the (unadjusted) differences identified in the preceding two figures large and statistically significant after accounting for potential confounders? To get a more accurate sense of the predictive power of various potential drivers of anti-tech attitudes, we estimate a regression with the YouGov data in figure 5 (and also other psychological predictors in figure S.5, using the Lucid data). We see that anti-establishment views and loneliness (plus a need for chaos and a need for uniqueness in the Lucid survey) are positively associated anti-tech attitudes.
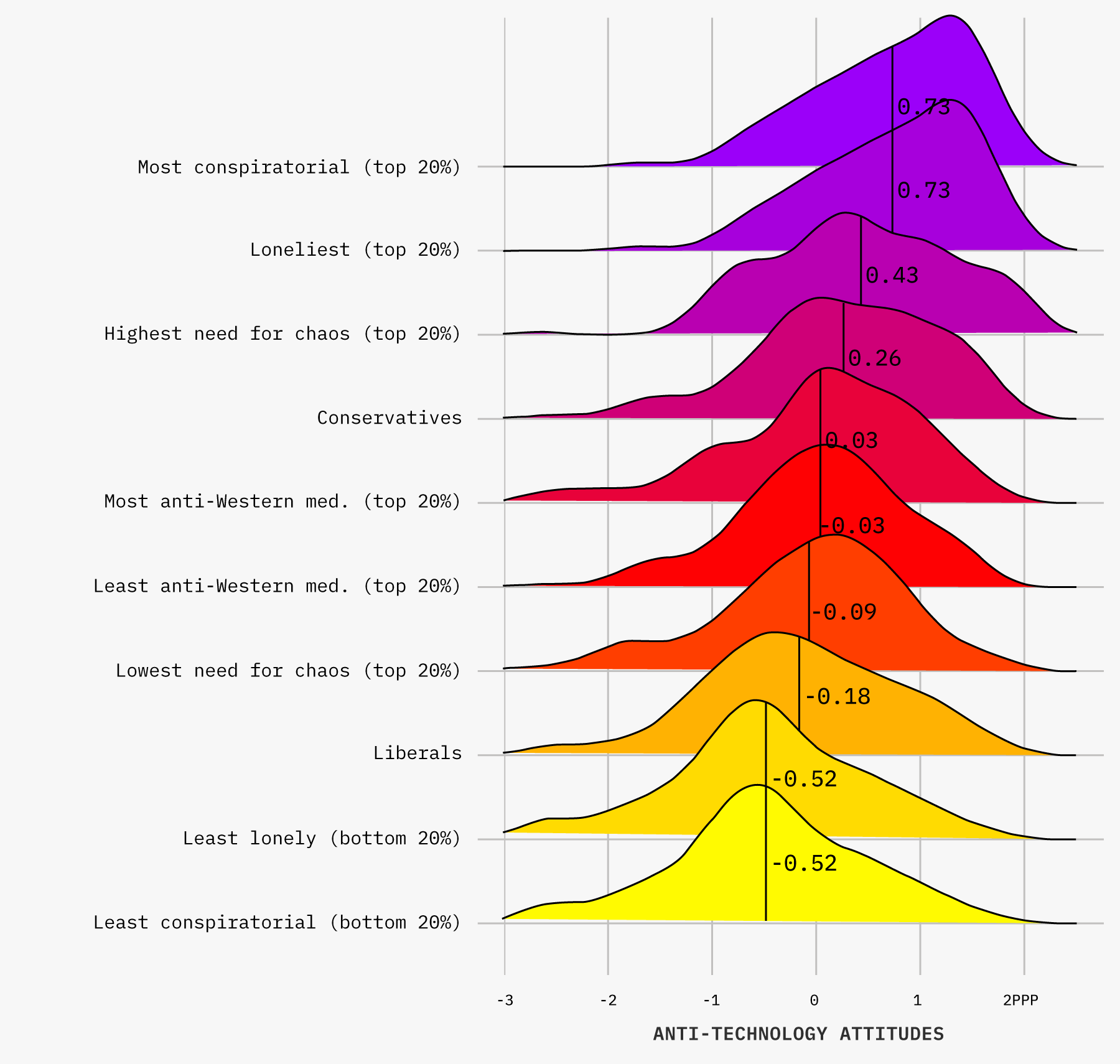
Conditioning on partisanship, age, education, race, gender, and church attendance (as well as loneliness and frequency of using modern technology), we see that a one standard deviation increase in conspiracism is associated with a 0.36 SD increase in anti-tech attitudes. The association between loneliness and anti-tech attitudes is smaller but still positive (a 0.14 SD increase in anti-tech attitudes is predicted for each 1 SD increase in loneliness). We also find that the partisan gap shrinks after adjusting for covariates (relative to the raw differences reported in figure 3), that older respondents are less skeptical of technology (even controlling for relevant covariates), and that more frequent use of modern tools and innovations is associated with less negative attitudes toward technology.
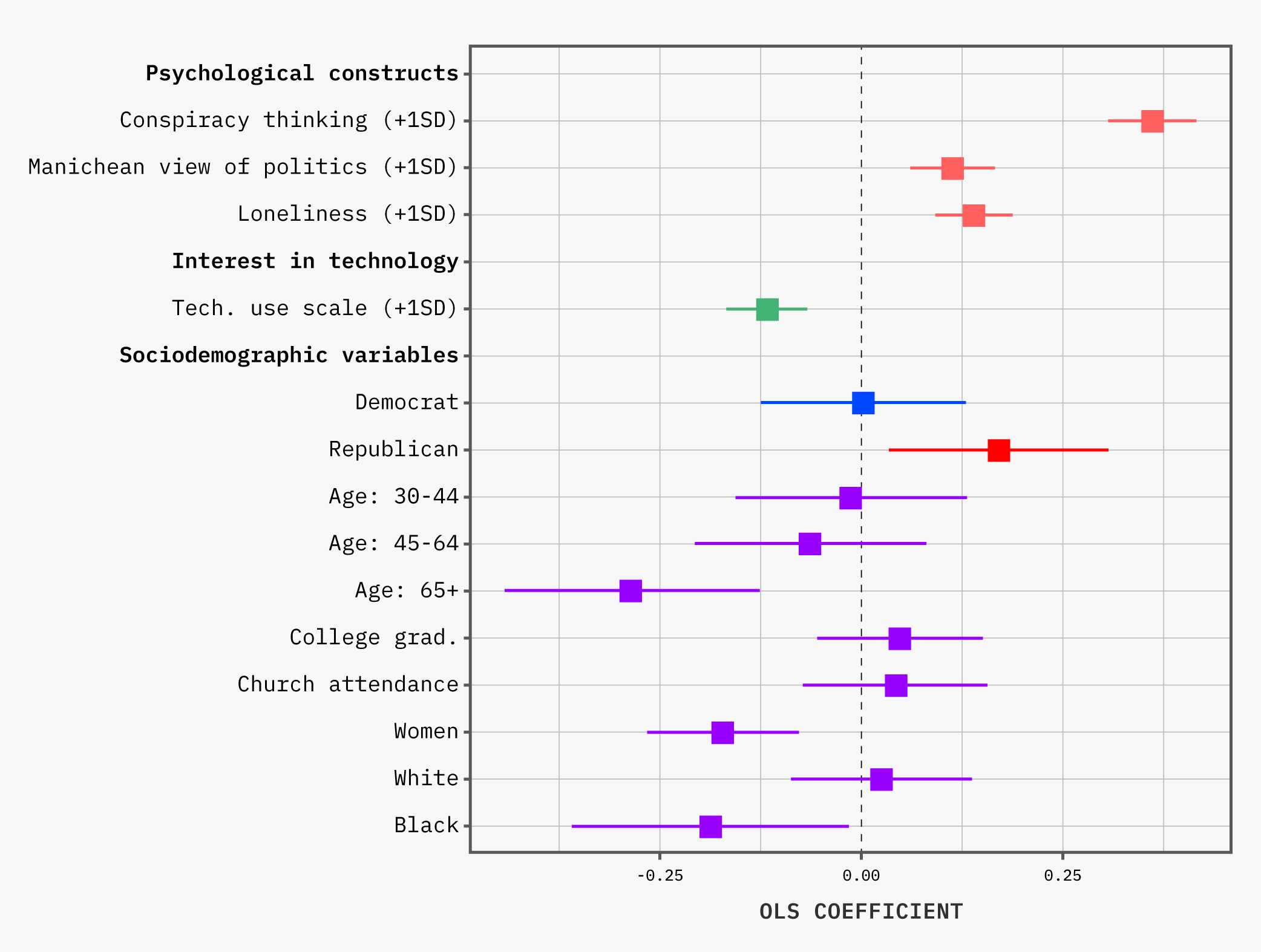
Next, we examine whether the size and direction of the positive coefficient on “Republican Party ID” (which some may view as a proxy for conservatism) change across the full range of values for conspiracism. As we saw, conspiracism is the best predictor of anti-tech attitudes, but does it matter differentially for Democratic versus Republican identifiers? To test this possibility, we interact partisanship with the level of conspiracy mindset, and otherwise maintain the same specification as the one displayed in figure 5. The inclusion of an interaction term allows us to assess whether the positive association between Republican Party ID and anti-tech attitudes is stable across levels of conspiracism, and we use the kernel regression approach proposed by Hainmueller, Mummolo, and Xu, which allows us to relax the assumption that the interaction between our two variables is linear. The results from this procedure are displayed in figure 6: we see that for the lower levels of conspiracy thinking, Republicans are no different in their anti-tech dispositions than independents. However, if a respondent exhibits average or above-average levels of conspiracism, then Republican Party ID is prognostic of elevated anti-tech views.
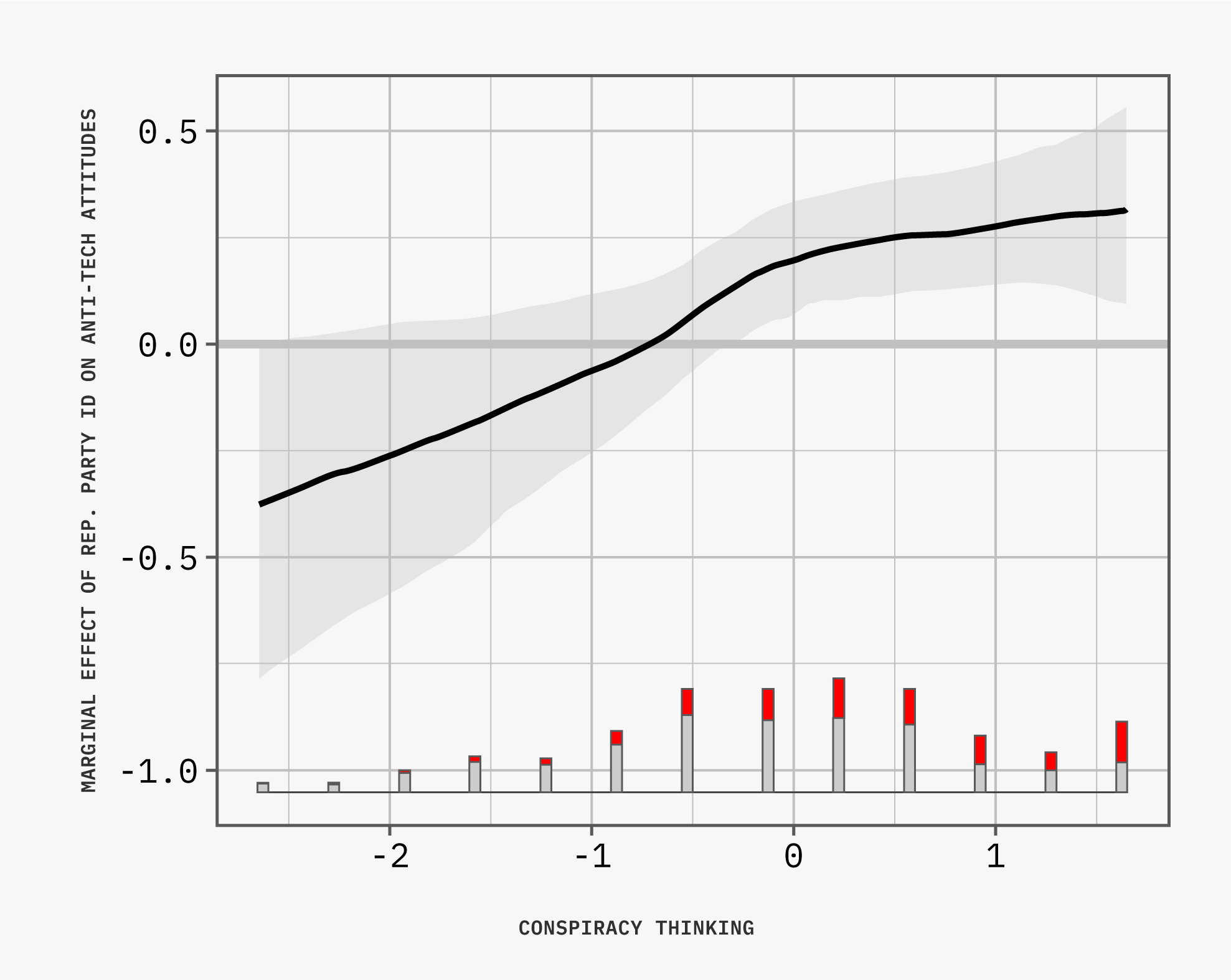
Study 2: Policy Preferences, Openness to
Innovations, and Extremist Behavior
Moving forward in our analysis, we focus on the relationship between anti-tech attitudes and policy preferences. We estimate two distinct models, setting the dependent variable to 1 if a respondent expresses support for breaking up Big Tech (model 1), or when a respondent agrees with the statement that the government should heavily regulate AI (model 2). Through these models, our aim is to uncover if, and to what extent, anti-tech attitudes influence public policy stances, especially when accounting for other potentially influential factors like political affiliation. We control for partisanship and other suspected confounding variables, ensuring (albeit imperfectly) that the observed associations between anti-tech attitudes and policy preferences are not merely artifacts of underlying political beliefs or unobserved factors like demographics, exposure to technology through frequent use, or certain psychological attributes and predispositions.
We see that a counterfactual one standard deviation increase in anti-tech attitudes is associated with an increase of 12.9 percentage points in support of breaking up Big Tech, whereas partisanship, anti-establishment orientation, and loneliness are not correlated with this policy preference. These and remaining conditional average marginal effects are displayed in figure 7.
The relationship between anti-tech attitudes and supporting regulation of AI is also positive and significant: we see an increase of 13.2 percentage points in the predicted probability of supporting regulation for a 1 SD increase in anti-tech attitudes, conditioning on demographics, partisanship, conspiracy thinking, loneliness, and the frequency of using technologies such as ChatGPT, smartwatches, smart home assistants, or social media. For this outcome variable, we see that Democrats are more supportive of regulation than independents, and that people who interact with the latest technology are not opposed to regulation (in fact, they are slightly more likely to support regulation of AI).
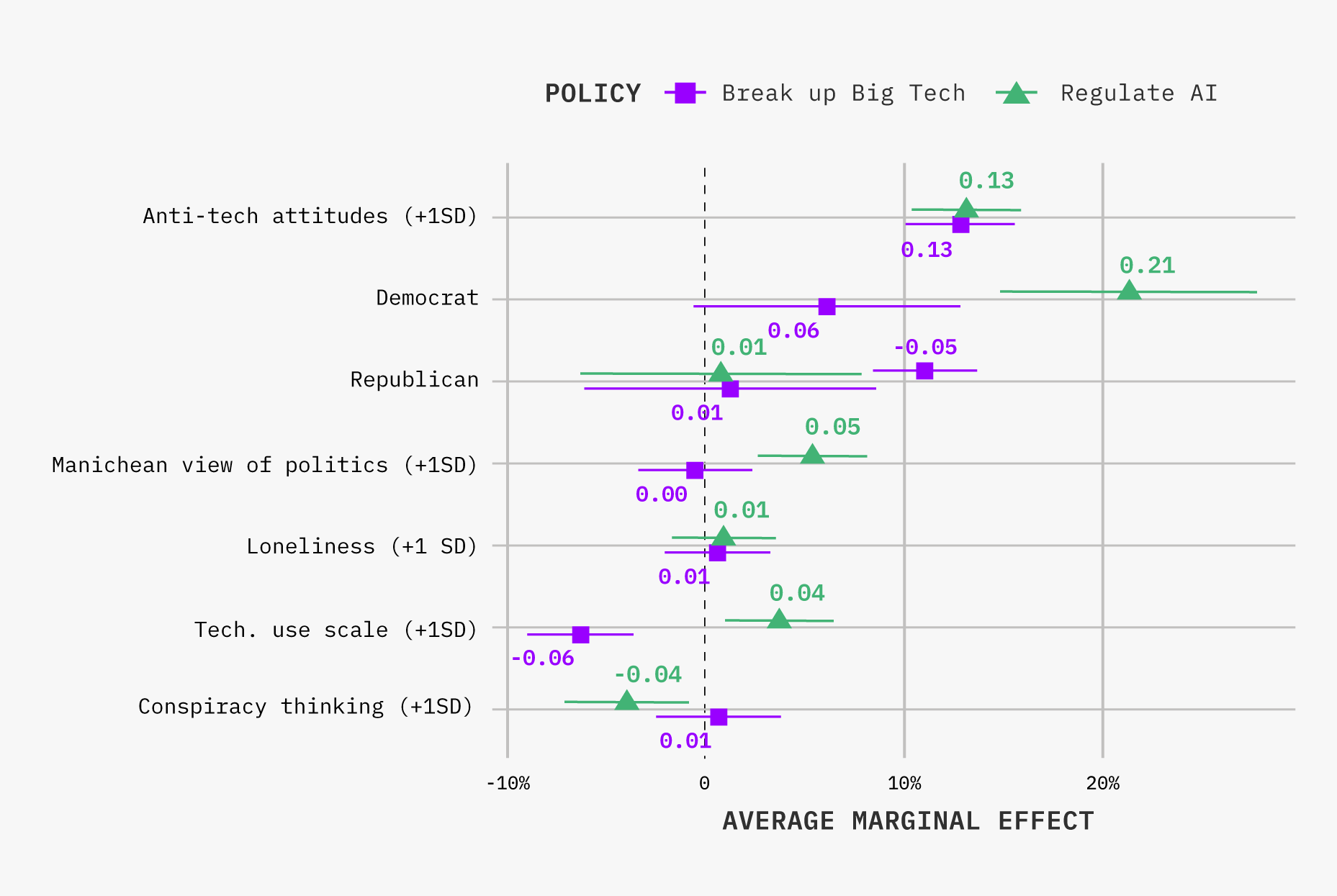
Setting the anti-tech attitudes to their maximum and keeping other covariates at their observed values for all respondents would yield an average predicted probability of regulation support of 84.7 percent. The same counterfactual exercise with anti-tech attitudes being set to their minimum value gives us an average predicted probability for 12.9 percent. That is, a hypothetical min-to-max. movement in anti-tech attitudes is estimated to produce an effect of 71.8 percentage points, which can be viewed as a massive effect.
Evaluations of Specific Technologies
Figure 8 presents predicted evaluations of five emerging technologies, capturing whether respondents view them as beneficial or detrimental to society. These predictions are based on logistic regression models that again control for key observables including partisanship, loneliness, conspiracism, Manichean worldview, technology interest and use, and demographics. The x-axis represents the full range of anti-tech attitudes, while the y-axis shows the probability of supporting each technology. Across all five technologies, we observe a consistent negative relationship between anti-tech attitudes and support for technological adoption. However, the strength of this relationship and the baseline level of support vary considerably across technologies.
Facial recognition for police use and automated misinformation detection by social media companies stand out as the most widely accepted technologies. Even among respondents with strong (top quartile) anti-tech attitudes, support for these technologies registered above 50 percent. For those with the most pro-tech attitudes, support exceeded 75 percent for both facial recognition and automated misinformation detection.
In contrast, the other three technologies— self-driving cars, brain chip implants, and robots replacing service workers—face much more skepticism. The steepest decline in support relative to anti-tech attitudes is observed for robots replacing cashiers, cleaners, and cooks. While individuals with the most pro-tech attitudes show about 50 percent support for this technology, this drops to nearly zero for those with the strongest anti-tech sentiments. Self-driving cars and brain chip implants for enhanced information processing face considerable skepticism in general. Notably, even among the most pro-tech respondents, the predicted probability of supporting self-driving cars barely approaches 50 percent. For those with strong anti-tech attitudes, support drops to around 15 percent or less.
Interestingly, the results suggest a divide between technologies that might be perceived as enhancing public safety or combating misinformation (facial recognition and automated fact-checking) and those that more directly replace human capabilities or alter the human body (service robots, self-driving cars, and brain implants). This divide persists across the spectrum of anti-tech attitudes, potentially indicating broader societal concerns about job displacement and bodily autonomy that transcend general attitudes toward technology. These findings highlight the nuanced nature of public opinion on emerging technologies: while general anti-tech attitudes are a strong predictor of opposition to new technologies, the baseline level of acceptance varies significantly depending on the specific application and its perceived societal impact.
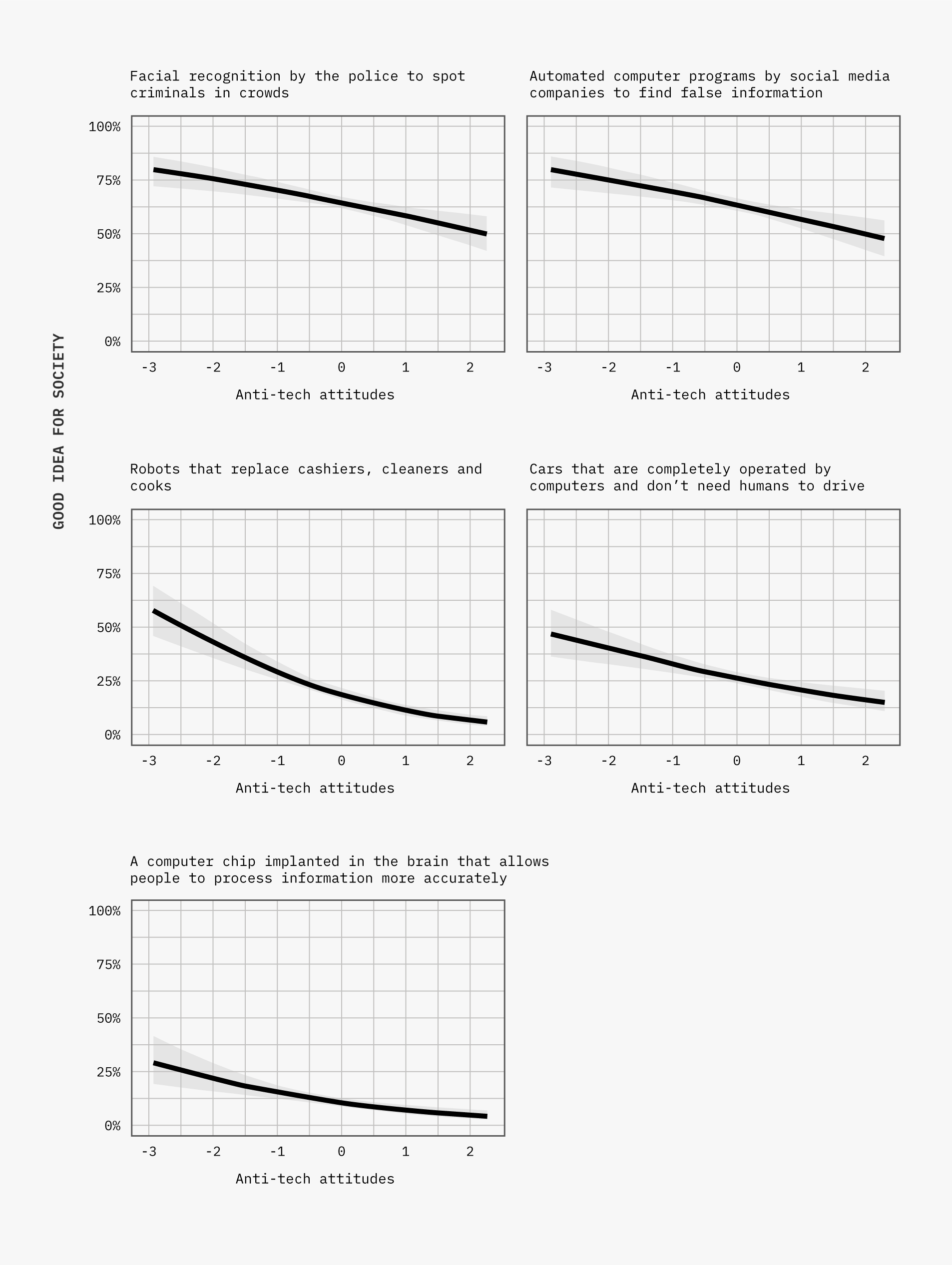
Approval of Politically Motivated Violence
Finally, we summarize our results from the firebomb vignette. We note that sympathy with the violent act was surprisingly high: 49 percent of respondents in dataset 1 agreed with “what the man said,” and 21 percent somewhat or strongly supported the action. A neutral option was provided when respondents were asked “Regardless of whether you agree or disagree with the man’s actions, how much do you agree or disagree with what the man said?” (five-point SA–SD scale, which has been dichotomized of the analysis that follows), but we forced respondents to take a stand on the man’s actions by using only a four-point scale (strongly support to stronly oppose) with no neutral option after asking “How much do you support or oppose the man’s actions?” We find that the probability of agreement with the sentiment of the letter (expressing the view that tech CEOs “just want to make money” and do not care if their products “divide our country, and hurt our children”) is 19 percentage points higher for each 1 SD increase in anti-tech attitudes (figure 9). The probability of agreement with the violent act itself does not rise with anti-tech attitudes, but it correlates positively with a need for chaos. Surprisingly, however, the need for chaos does not correlate with the weaker type of support (agreeing with the letter) in the expected direction.
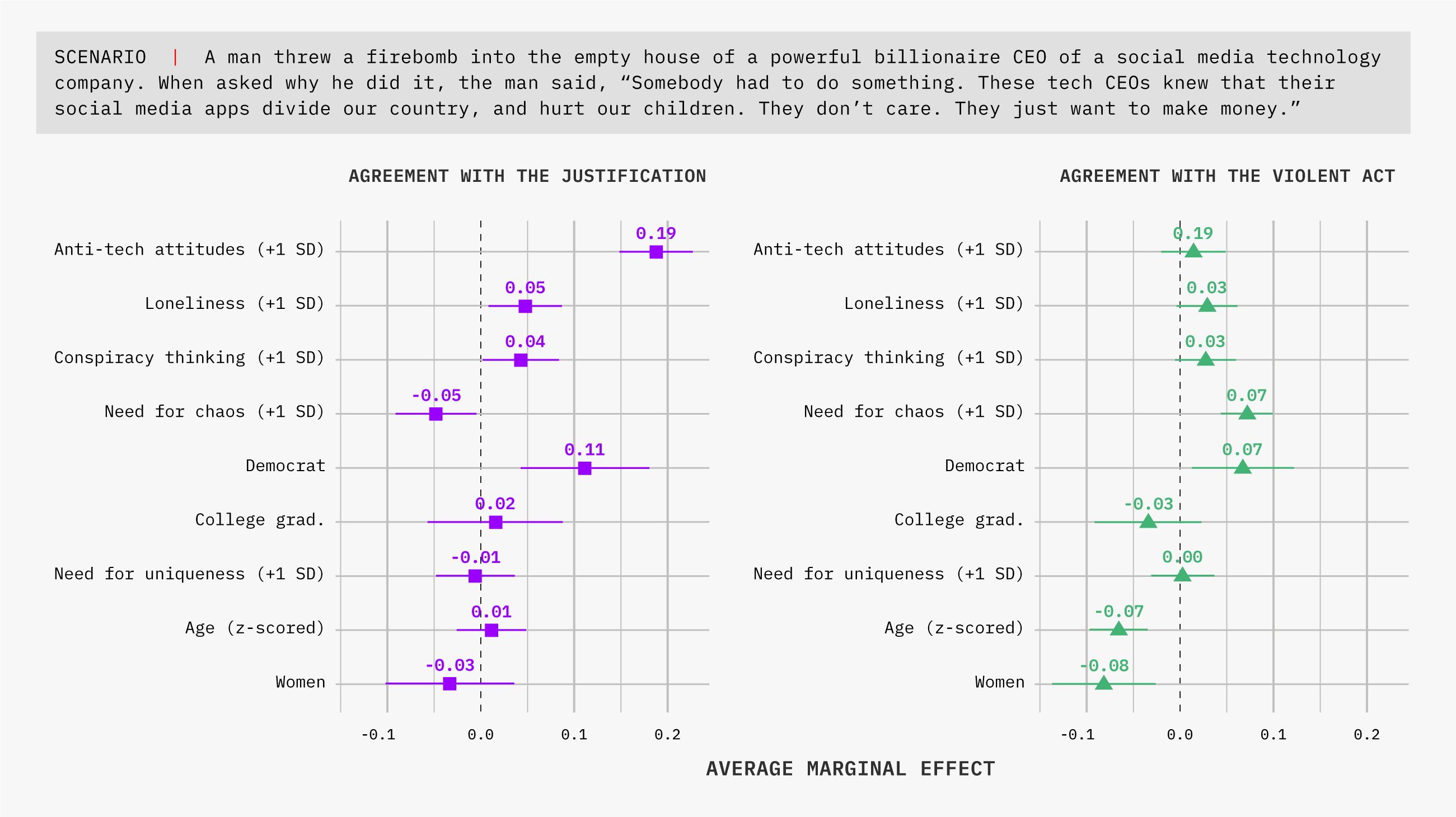
Study 3: Persuasiveness of Arguments about AI
Studies 1 and 2 focus on the construction and measurement of anti-tech orientation. But a separate question is whether individuals can be persuaded about technology: are anti-tech perceptions fixed, or are there certain types of arguments that push the public to be more or less receptive? These questions are of particular import, since recent research finds that even amongst experts there are large disagreements over the risks of future technology like AI. In this study, we focus on arguments for and against AI, a relatively new technology. Each of our YouGov respondents was asked to evaluate three pairs of arguments about artificial intelligence. A sample screenshot is shown in figure 10 (we followed the approach proposed by Blumenau and Lauderdale).
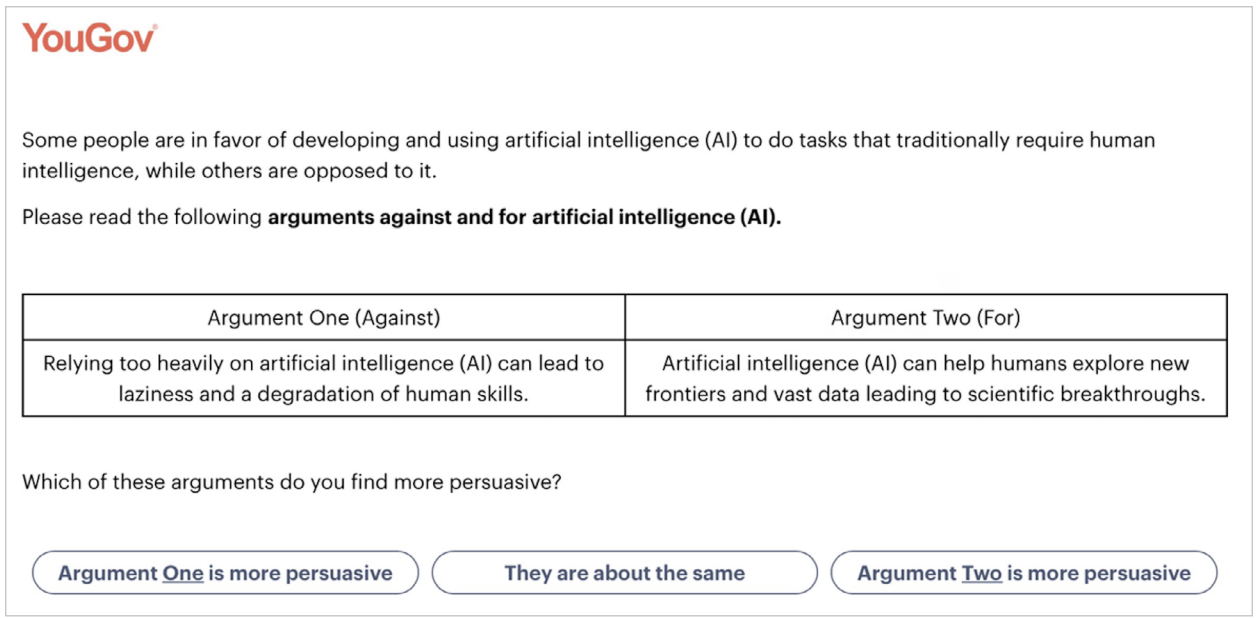
Our dataset contains 8,100 judgments (from 1,350 US respondents) about ten arguments (five pro-AI and five against AI). Participants were randomly shown two arguments—one that argued in favor of AI and the other argued against it. They then indicated which of the two (randomly paired) arguments they considered more persuasive, or whether they considered them to be equally persuasive. All arguments that a respondent could potentially evaluate are listed and ranked by their raw win rate in figure 11. We see that, on average, arguments against adopting AI were slightly more persuasive than pro-AI arguments. However, the most successful argument of all was a pro-AI argument that said, “AI can speed up medical research and improve early diagnosis of diseases.”
A separate analysis is provided in the SI (figure S.11), where we estimate the strengths of arguments while controlling for the relative strengths of the counter-arguments they were facing, using a Bradley-Terry model. The results are substantively unchanged: the top three and the bottom three strongest or weakest arguments respectively are the same (and there are small movements in the relative rankings of the arguments of medium strength).
The conclusion from this experimental study of persuasiveness is that people remain skeptical about new technologies like AI. But because these innovations are new (and in some cases hypothetical), we also show that citizens are somewhat persuadable. Emphasis on the positive medical benefits or replacement of repetitive tasks makes people more amenable to AI. But concerns about misinformation, replacement of human jobs, and more general degradation of human capacity makes people more skeptical.
Conclusion
We fielded a pair of surveys and a survey experiment on persuasiveness to measure Americans’ attitudes toward several key questions related to technology. For instance, do Americans attribute certain societal problems, such divisive politics, feelings of loneliness, and the propagation of hate and misinformation to the influence of social media platforms? Do citizens feel threatened by the coming AI revolution? These questions are particularly important as AI technologies may increasingly become part of people’s daily lives. Moving beyond specific domains, is there a general anti-tech orientation? Aggregating our survey instruments to quantify broader anti-technology sentiments, we found that the first factor that emerged corresponded to a general, critical attitude toward modern technology (not limited to social media apps).
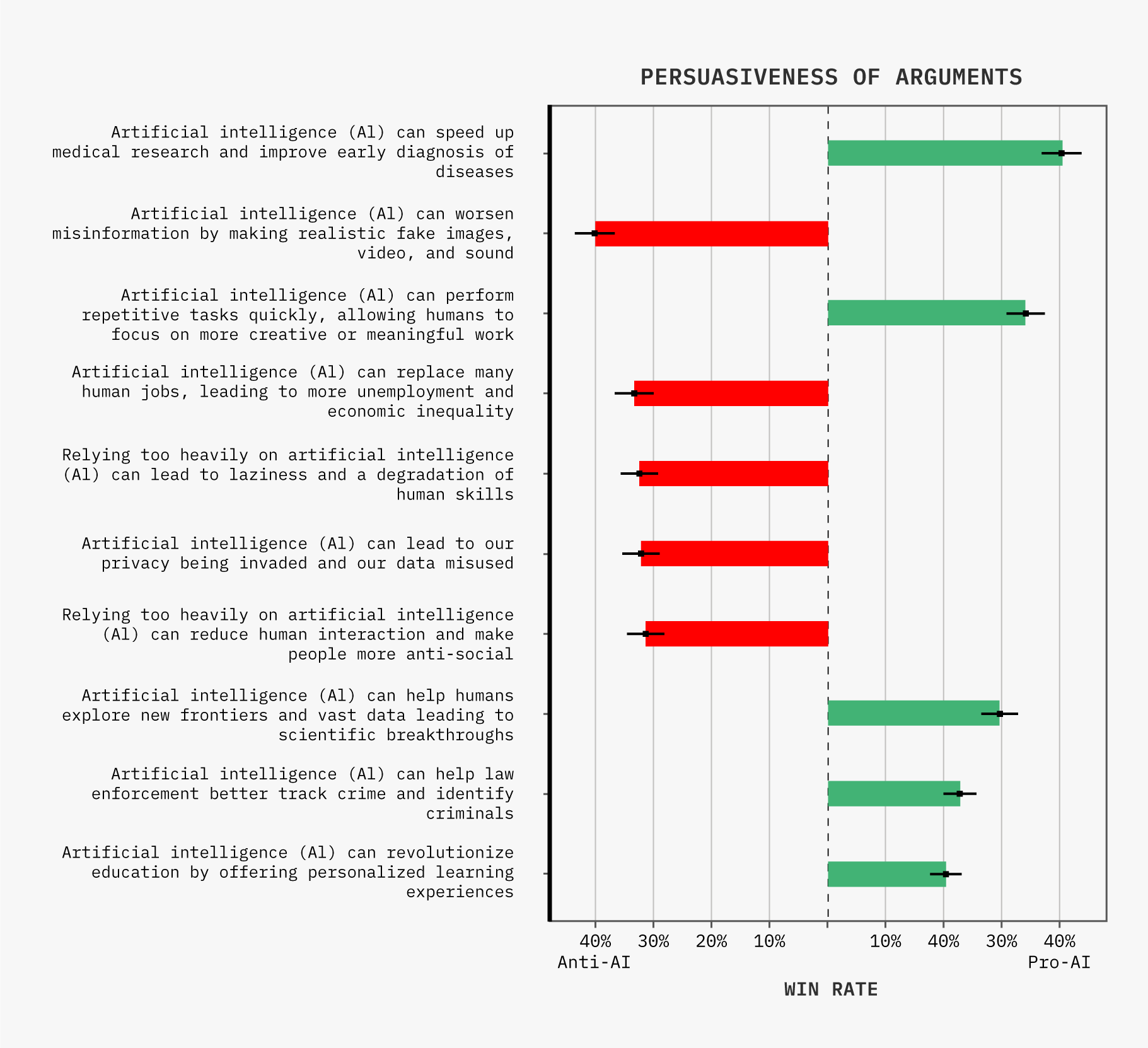
Our findings provide several insights about the nature and implications of anti-technology attitudes in the United States. We find that anti-tech sentiment is a coherent orientation, distinct from but related to other psychological and political factors. This orientation strongly predicts policy preferences and, in some cases, support for extreme actions. We identified which segments of the population were most likely to score high on our anti-tech orientation, finding that loneliness, conspiracy thinking, need for uniqueness, and need for chaos correlate with the general anti-technology factor (these relationships hold after controlling for partisanship and education). Finally, beyond personal dispositions, we show that anti-tech sentiments shape respondents’ policy preferences, especially concerning the governance and regulation of technology. The paper also documents how anti-tech attitudes might breed acceptance or even endorsement of extremist actions, including support for violence against tech businesses or technological entities (or their proponents), establishing that there is a clear association between anti-tech beliefs and supporting justifications of violent actions against the leaders of tech companies. Finally, we show that the public can (currently) be persuaded by both pro- and anti-AI arguments, although anti-AI arguments were on average deemed more persuasive.
With these results in mind, what are the implications for theory, policy, and future research? Anti-tech attitudes represent a coherent orientation that is not purely explained by partisanship, suggesting that its roots may be more psychological than ideological. Given increasing criticisms of technology companies by certain politicians and elites, and given attempts to regulate social media and AI companies, anti-tech sentiment will likely remain a politically salient issue, possibly cutting across traditional party lines. This growing salience has led to increased scholarly attention on the role of technology companies in governance and society.
For instance, Cupać, Schopmans, and Tuncer-Ebetürk have cautioned that “technology corporations have emerged as a new quasi-governing class that holds political power without democratic legitimacy,” and Culpepper and Thelen have said that “[f]irms with platform power benefit from a deference from policymakers.” The noted decrease in state power extends to labor power as well; using perhaps deliberately loaded and provocative language, the dependence of some workers on digital platforms has been described as techno-feudalism, a concept related to concerns about “platformization” and surveillance. Furthermore, as other research has shown, entrepreneurs in the technology sector and other elites that are pro-technology and cheering the arrival of AI have very different preferences and attitudes than other citizens.
Concerns about technology are likely to grow if deepfakes, misinformation, and AI-generated ads play increasing roles in political campaigns. Policymakers and technology companies would do well to place guardrails on such use. That said, perceptions among both citizens and regulators about the effects of digital media on democracy may not be in line with the existing available research. As Budak et al. note, “sweeping claims about the effects of exposure to false content online … are inconsistent with much of the current empirical evidence.” This discrepancy between public perception and scientific evidence highlights the need for continued research and effective communication of scientific findings to the public and policymakers.
To the extent that dangers of digital media or new apps may be exaggerated (or the benefits underappreciated) by citizens, it is possible that informational interventions could correct misperceptions or that intuition about fairness (e.g., fairness of automation) could be adjusted via framing. And, as our pairwise experiment suggests, supporters of new technology may wish to emphasize and explore the positive arguments for AI—for example, that AI can improve medical care and disease diagnoses—while addressing negative concerns of the public, namely increased misinformation and job loss due to automation. While these strategic communication approaches may help shape public opinion, it is crucial to ground such efforts in a thorough understanding of existing anti-technology sentiments and their implications. At the same time, the welfare effects of new technologies are a subject of continued study, and future work needs to grapple with both direct and subtle network effects and consumption spillovers (e.g., users demand payment to stop using apps like Instagram and TikTok, but they would simultaneously be willing to pay for an outcome where everyone de-activated these apps, according to incentivized experiments by Bursztyn et al.).
As e-commerce, ride-sharing, online dating, cryptocurrency trading, the Internet of things (network of physical objects embedded with sensors), ubiquitous computing, applications of AI, and other technologies continue to emerge and reshape the economy, anti-tech sentiment will play an increasing role in politics. How politicians seek to mobilize supporters with anti-tech appeals, and whether it begins to emerge as a partisan issue remain important future questions; but our study advances our understanding of the pre-existing reservoir of anti-tech sentiment by providing a comprehensive examination of anti-tech attitudes, their correlates, and their potential consequences. We have shown that anti-tech sentiment is a coherent orientation with significant implications for policy preferences and potential support for contentious actions. Future work should continue to explore the evolution of anti-tech attitudes over time, these attitudes’ relationship to emerging technologies, and their impact on political behavior and policy outcomes.